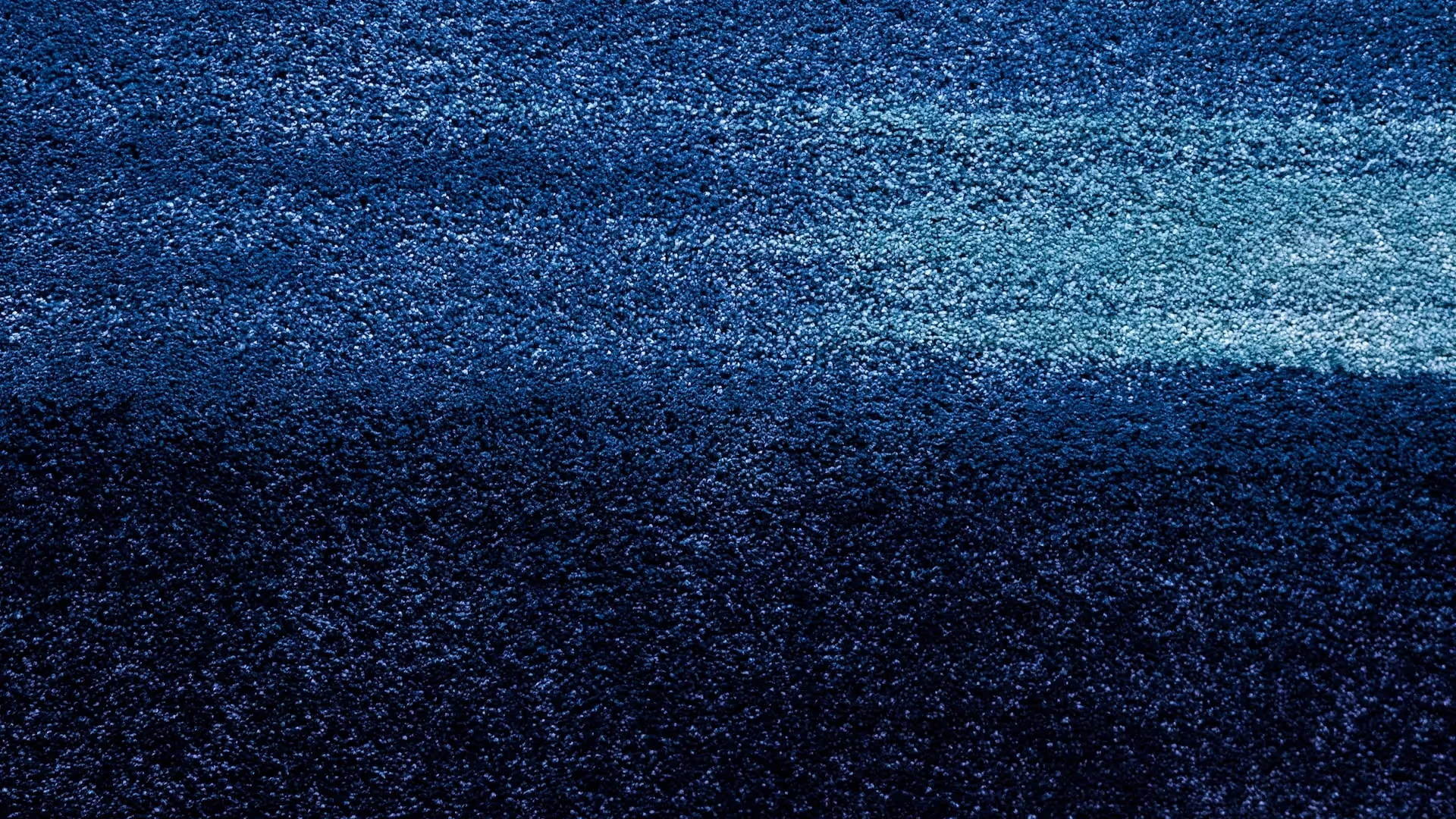
Once upon a time, Gartner predicted that 40% of all data science tasks would be automated. Naturally, this caused some discussion about the future of the profession and whether it would still be the hottest position on the market. However, as the field progresses, we’re finding out that the question of automation isn’t so straightforward. In fact, automation may not even be the most exciting piece of the data science journey. Gartner didn’t get it wrong, per se, but they may have missed an important step.
Gartner’s Predictions Were Based on a Highly Technical, Competitive Field
Data science is still technical. But back in 2017, when Gartner’s report came out, the field was undergoing a transformation. IT teams still built pipelines to answer specific business-related questions and provided the insights to business teams, but business users were interested in analyzing data themselves to speed up decision making.
Domain experts helped bridge the gap between the business set and the IT department, but companies needed faster insights. Why not train other departments to engage in data science tasks? The market for tools that would automate aspects of data science requiring technical expertise began to grow.
Citizen data scientists were already using self-service BI tools, but companies were interested in moving into more complex tasks still dominated by trained data scientists. Automation would allow business users to engage in sophisticated analysis and take advantage of real-time decision-making.
In addition, the competitive field of data science meant businesses competed for talent and needed to make do with fewer data scientists on their teams. This meant teams spent a lot of time on mundane tasks like troubleshooting and maintenance, finding appropriate data, and waiting for pipelines to be built.
Automation would mean two things:
- Data science teams (sometimes consisting of a team of one) could make headway in data analysis faster by offloading some of the simpler work to business teams.
- Citizen data scientists could take on data science tasks explicitly related to their department, which would give them more independence and allow faster pursuit of new ideas.
And according to Gartner, this would happen in just a matter of a few years.
So Did These Predictions Come True?
The short answer is: sort of. More tools than ever are on the market to help automate data science tasks, but data scientists continue to spend a lot of time on the same mundane tasks. At the same time, citizen data scientists still aren’t self-sufficient enough to achieve the vision presented. Companies continue to hold valuable data in stasis without ever using it. So, what happened?
The automation question is more complex. Many focused on whether the data science field would cease to need people and instead rely only on the machines they built. This misses a big question.
Where Is Automation Most Appropriate?
It’s not just about taking tasks off the plate of data scientists. Instead, automation is also about making those tasks more efficient in order to keep up with the speed at which insights should happen. The pandemic made it clear that models built on data from months ago weren’t going to work for the greater business good anymore. Companies needed to efficiently screen the right data for the right insight at the right time — quickly, right now.
Automation can make data analysis a more important part of business, but it can also propagate human error without a strong data science foundation underneath. As businesses pursue automation, they’ve often created more complexity because:
- The tools they introduce don’t always play nicely with each other, causing integration issues and security loopholes.
- Business users have introduced their own tools to help manage tasks, increasing shadow IT loads.
- Infrastructure challenges — including migration to the cloud — have made it difficult to maintain observability over tools and usage.
Ultimately, Gartner’s prediction hasn’t quite come to pass just yet. Although much of data science has become automated, it’s created more challenges in its wake. For example, being able to generate a lot more models with the same resources leads to new challenges tied to scaling the management, maintenance, and governance of those models.
Companies Need an Operational Layer to Ensure Automation Is Effective
To ensure that automation reduces the workload data scientists face and enables citizen data scientists like Gartner predicted, companies need a new data management paradigm. A data operating system is an end-to-end solution that connects all tools and data sources within the company’s ecosystem.
When companies onboard new tools, there is a resource overhead to train employees and ensure integration and a maintenance overhead to keep these tools running in peak form. Many of these integrations become fragile and lead to silos that prevent accurate data insights. An operational layer provided by a data operating system can connect these tools seamlessly with little disruption to business operations.
The data operating system then automates tasks that take up time from data scientists — cleaning, maintenance, observability, and even building pipelines for business users. It frees them up to engage in higher-order tasks and ensures a high level of security for data assets.
A business-oriented operating system lessens the complexity of data science for business users. For example, someone from the marketing department wouldn’t need to wait for permission to use certain data columns and rows or for the data science department to build the correct pipeline. They would be able to:
- See what data is available through a self-service portal.
- Access it through a cross-platform attribute-based governance system.
- Drag and drop the data elements they need to build an appropriate pipeline, when a simple pipeline will suffice, without coding knowledge.
A Data Operating System Helps Companies Achieve What Gartner Predicted
A data operating system ensures companies can automate data science tasks and receive value back. The current state finds automation creating more challenges, but it doesn’t have to be this way. When a company implements a true data operating system, they can automate the tasks that matter while facilitating the data tasks that lead to value.
DataOS from The Modern Data Company is the world’s first data operating system. It’s designed to integrate seamlessly with all apps, tools, and legacy systems to bring clarity to your data ecosystem. It’s business user-friendly while offering the high-level tools data science teams need to execute in-depth projects