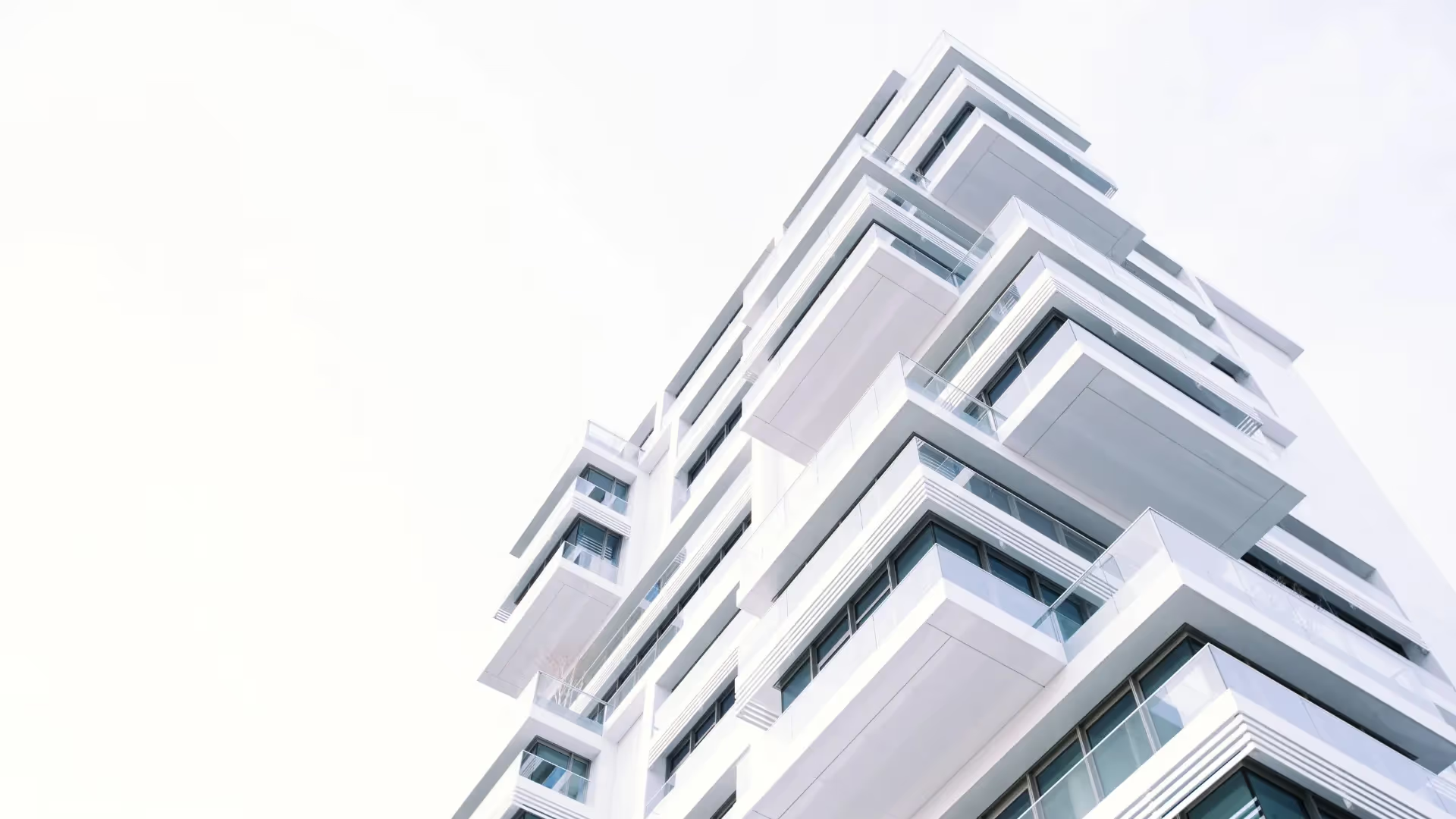
There has been tremendous growth in demand for and acceptance of advanced analytics, data science, and artificial intelligence over the past few years. While this progress will be a great thing in the long run, it has also eclipsed companies’ abilities to effectively scale their current approaches for developing and deploying analytics processes. The fast-expanding adoption of DataOps is one way that companies are trying to enable their scale to meet their demand. In this blog post, we’ll look at why DataOps is needed, what DataOps is, and how implementing DataOps successfully within an organization adds value.
Why DataOps Is Needed
For many years, companies have struggled to unlock the full potential of analytics. One big cause of this issue is the inefficiency and lack of repeatability of traditional analytical process development and deployment methods. For example, it is widely accepted in the field – even today –that between 70% and 80% of time spent developing advanced analytics processes is still spent acquiring, cleaning, and wrangling data. To outsiders those numbers might seem shocking, but it is an unavoidable consequence of companies managing their data in ways that are not friendly to advanced algorithms and complex computational requirements.
Another major inhibitor of further progress is the often-painful, inefficient, and time-consuming procedures in place for deploying analytical processes once they are built. In many cases, a lot of custom work is required to take a proven prototype and deploy it into operational systems so that the process can be run at scale. Messy handoffs between the analytics team that builds the processes and the IT team that deploys them are made worse by the fact that advanced approaches like artificial intelligence push the limits of what today’s systems can handle. The combination of unusual complexity paired with massive processing requirements strains all aspects of deployment and management to their limits.
These same processes, once deployed, are often not documented well enough for long-term support purposes and can require substantive manual intervention to address the inevitable bugs or desired upgrades that are identified. The analytics team that builds processes also typically can’t escape being an integral part of the ongoing management of those processes. This means that as more successful processes are completed, there is a higher and higher percentage of time spent maintaining and managing existing processes and a lower and lower percentage of time spent creating new and innovative processes that will drive value. This is frustrating and demoralizing for analytics organizations while simultaneously being a misuse of high value – and expensive – resources by the company.
What Is DataOps?
DataOps is aimed at helping companies derive more value, faster from their advanced analytics initiatives by making the development, deployment, and management of analytics processes more standardized, automated, and scalable. It is a set of process-oriented methodologies that can take full advantage of the latest available technologies in combination with people who are open to changing some of their traditional ways of working.
DataOps focuses on automating much of the testing, monitoring, and maintenance of a process so that less time is required on all fronts. It borrows heavily from agile methods and DevOps approaches in order to combat the unusual requirements of advanced analytics processes. In a traditional DevOps environment, most of the processes being deployed and managed are fairly standard in their processing requirements, complexity, and consistency. With advanced analytics, these processes are much more fluid. In fact, many advanced analytics processes update themselves over time. This means that what works best for a process or set of processes today may not be best tomorrow.
This is where agile methodologies come into play. By incorporating agile, DataOps recognizes the need for flexibility and rapid adaptability that goes beyond what most DevOps environments require. The rules in place are kept to a minimum so that adjustments can be made. These adjustments, of course, come with risks and implications of their own. But by following an agile approach, DataOps teams can tackle challenges quickly and incrementally. However, there is no doubt that DataOps is a difficult and complex approach to implement.
In the end, DataOps implemented properly can help streamline the core phases of the analytical development process. This includes 1) making the upfront data phases more efficient, 2) better standardizing the development phase, 3) streamlining the deployment phase, and then 4) automating the ongoing monitoring and maintenance phase. A typical analytical process flow can be seen in Figure 1.
Figure 1. A typical analytical process flow
The Benefits of Implementing DataOps
Implementing a DataOps team, platform, and philosophy within your organization will not be an easy task. Multiple teams that focus on distinct but interconnected disciplines will have to come together and coordinate effectively to make DataOps become a reality. This includes – among others – the core skills and people within the analytics and data science team, the data engineering team, and the IT and systems team. Each team must ensure that their needs are met, and each will be impacted by the DataOps processes and technologies that are implemented.
As discussed previously, even if your organization already has a robust DevOps capability, it will take significant work to implement DataOps. This is due to two primary causes: first, analytical processes are often more complex and less rigid than the typical processing managed by a DevOps environment. These differences need to be accounted for. Second, tools to support DevOps are evolving rapidly, and there are some good solutions available to get you started. The same is true for DataOps, but it is further behind on the maturity scale. As a result, you can expect more customization and bespoke development to get a DataOps solution implemented in the near future. Over time, as DataOps matures, this issue will lesson.
All the hard work of implementation can pay off in the end from a variety of angles, however. Having standardized data pipelines will make new processes more consistent and lessen the chance of major bugs. This also allows for more rapid development of new analytics processes. At the same time, those building an analytics process will be aware of the standards they need to follow as they build, which will lead to more transparency and consistency across processes. Cataloging each model and its purpose, as well as tracking changes made to it over time, helps tremendously with identifying outdated processes and enforcing governance standards. Finally, having automated processes to monitor and assess data quality and integrity along with analytical process output provides the ability to catch problems early.
If your organization has increasing demands for analytics and is struggling to scale what you’ve got, you shouldn’t be asking if you need DataOps today. Rather, you should be focused on how to get started implementing DataOps right away. DataOps is rapidly going mainstream and will be a critical component of any organization’s efforts to better scale, govern, and automate analytical processes.
Learn more about The Modern Data Company by visiting our website.
Contact us to find out more about DataOS capabilities or to schedule a demo.