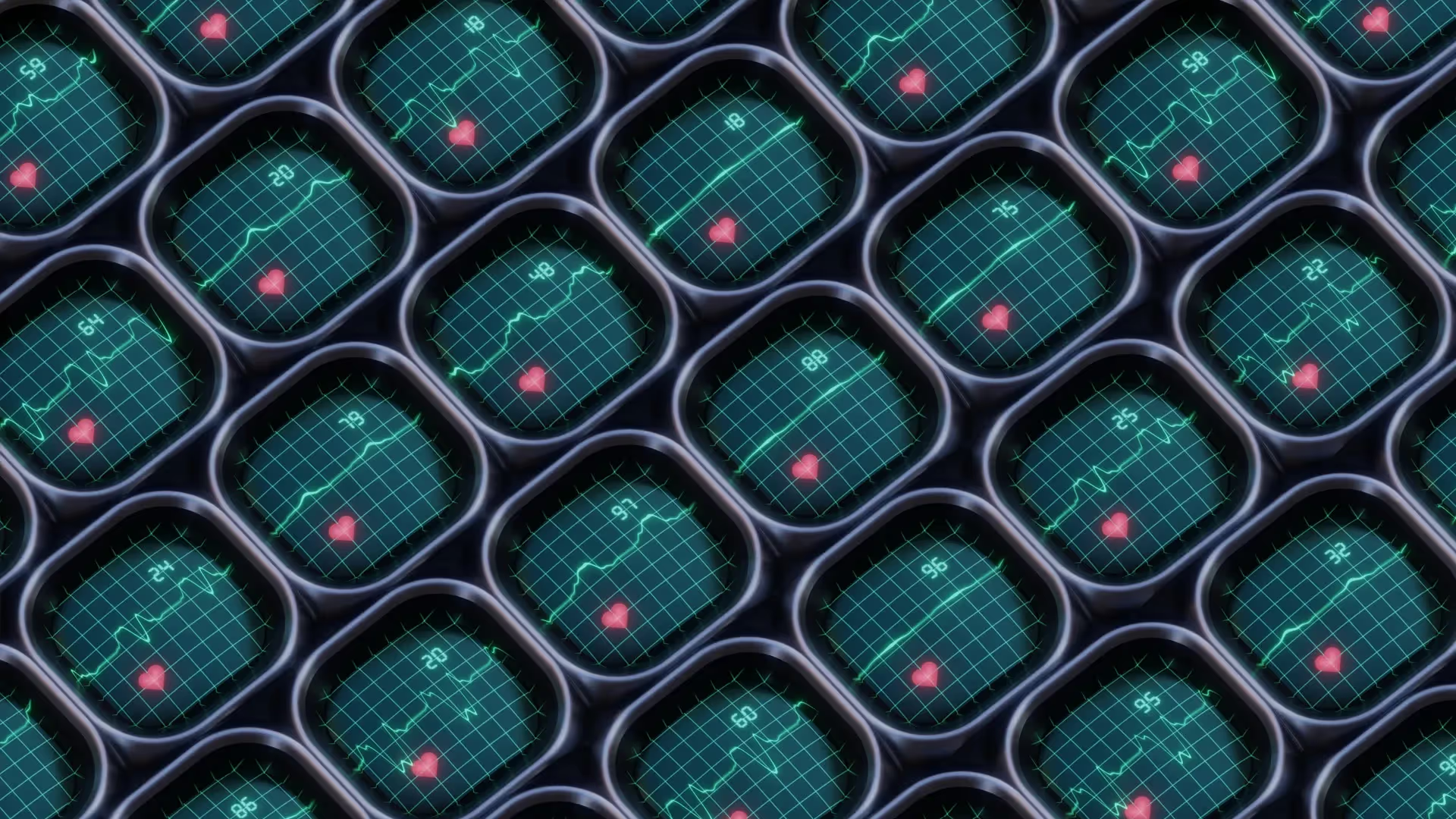
Prevention and early intervention are essential to building an effective healthcare approach that supports patients from start to finish. The critical component of this approach is predictive analytics — analyzing big data gathered from patients, consumers, and research to provide actionable insights about a patient's current and future healthcare needs. Although this is the ideal approach, many healthcare organizations struggle to operationalize their data and see results from new technology investments.
DataOS can address all these challenges. Let's explore how healthcare organizations can utilize a data operating system to unlock the value of healthcare data and provide truly remarkable service to patients.
Healthcare Must Integrate Predictive Analytics into Operations
Healthcare relies on a variety of data types, including:
- Clinical analytics - analyzing patient data to improve patient outcomes and quality of care
- Financial analytics - using data to optimize revenue, control costs, and manage financial performance
- Operational analytics - improving the efficacy of healthcare operations
- Population health analytics - analyzing population data to identify and address public health issues and improve outcomes
- Supply chain analytics - optimizing the procurement, distribution, and use of medical supplies and equipment
The challenge with analytics like these is creating a data ecosystem that supports real-time data processing without significant burden or cost to the already thin margins of most healthcare organizations. Additionally, a lack of technical expertise may leave smaller hospitals and healthcare centers struggling to implement the types of analytics patients expect. And while patients may not know anything about analytics, they know when doctors can monitor their symptoms and implement treatments early.
What's Keeping Healthcare Organizations from Leveraging Predictive Analytics?
Healthcare organizations often struggle to implement predictive analytics for a variety of reasons, even with heavy investments in new technology solutions and services. What follows are some of the biggest obstacles.
Data Silos
Healthcare organizations have disparate data sources and formats, making gathering and integrating data for analysis challenging. At this point, it's also highly likely that past technology investments and legacy systems further contribute to the breakdown in integration.
Silos can limit data visibility across an organization, preventing healthcare providers from having a complete and up-to-date view of a patient's health history. They can make accessing data from multiple sources difficult, resulting in duplication of effort and reduced efficiency. In the best-case scenario, they can lead to missing or inconsistent data, which can negatively impact the accuracy and reliability of analytics and undermine success in patient care. In the worst case, data silos can make it challenging to comply with regulations such as the Health Insurance Portability and Accountability Act (HIPAA), which require secure and consistent data sharing between different systems and departments.
Technical Expertise
Implementing predictive analytics requires specialized technical skills, but healthcare organizations may not have personnel with the necessary technical skills to implement and maintain analytics solutions. The market's technical talent shortage and the high demand for analytics experts can make it difficult for healthcare organizations to find and retain the in-house expertise they need to design, deploy, and maintain cutting-edge data solutions.
Additionally, healthcare data can be complex and diverse, requiring specialized tools, apps, and services to process and analyze effectively. The fast pace of technological change in analytics can make it difficult for healthcare organizations to keep up with the latest developments and implement these new solutions effectively. Making it worse, implementing analytics solutions in a healthcare organization can be challenging due to the need to integrate with existing systems that have their own technical requirements, such as electronic health records (EHRs).
Resistance to Change
Healthcare organizations can be slow to adopt new technologies. Healthcare workers are often used to working with familiar tools and processes that have been in place for many years, and changing these can be challenging due to resistance from staff and stakeholders. New technologies can be perceived as complex and challenging to understand, creating fear and resistance among healthcare workers. It's difficult enough to try to decommission legacy systems from an operational standpoint; when the people aren't on board either, it's nearly impossible.
Privacy and Security Concerns
Healthcare data is some of the most sensitive personal information that exists. If this information were to be disclosed or used without authorization, it could have significant consequences for the individual. The unauthorized release of healthcare information could violate regulations such as the HIPAA in the United States, which sets standards for protecting sensitive medical information.
Healthcare organizations must implement strict privacy and security measures to protect patient health information. These protections include processes for securely storing, transmitting, and analyzing data and ensuring that only authorized individuals have access to it. Furthermore, healthcare organizations must also consider privacy when designing and deploying healthcare analytics systems and applications. These measures include incorporating privacy-preserving technologies, such as data anonymization and privacy-enhancing algorithms, to minimize the risk of privacy breaches while enabling valuable insights gleaned from the data. With currently common technology solutions, this is a nearly impossible balance.
Budget Constraints
Predictive analytics can be expensive to implement and maintain, and many healthcare organizations have limited budgets for technology investments. They must prioritize spending based on their most pressing needs. Modernizing technology must compete for a share of the budget with other important initiatives, such as hiring additional staff, purchasing new medical equipment, or improving facilities.
If the hospital or healthcare center relies on public funds, budget constraints may further limit the money available for modernizing technology. Additionally, the lack of private funding sources earmarked for technology upgrades in the healthcare sector can make securing the resources needed to implement new solutions challenging.
A Data Operating System Smooths Obstacles and Launches Predictive Analytics
Despite the challenges, many healthcare organizations are finding the benefits of predictive analytics, such as improved patient outcomes and cost savings, make the investment worthwhile. Healthcare organizations need a single solution to streamline and simplify data, protect it, and make it readily available for IT and non-technical users.
DataOS is an out-of-the-box operating system that integrates critical healthcare tools and data sources into a single platform to make predictive analytics possible. It enables:
- Data integration: DataOS provides a centralized platform for integrating and managing disparate data gathered from multiple sources, such as EHRs, imaging systems, lab results, and demographic data. A centralized platform gives healthcare organizations a comprehensive view of patient data, essential for effective predictive analytics.
- Data governance: DataOS offers native data governance and management based on attribute-based access controls. This helps healthcare organizations maintain the integrity and reliability of the data while also enabling self-service for users.
- Scalability: The platform provides a scalable infrastructure that can handle the growing volume, variety, and velocity of data generated in healthcare. This capability allows healthcare organizations to handle large and complex data sets, integrate multiple tools now and in the future, and operationalize data for real-time decision making.
- Real-time processing: DataOS enables real-time processing of data, which is crucial for predicting patient outcomes in real time. This speed allows healthcare organizations to make timely and informed decisions about patient care and improve overall patient outcomes.
- Collaboration: DataOS facilitates collaboration among healthcare professionals, researchers, and data scientists. Seamless collaboration helps healthcare organizations leverage different professional expertise to generate meaningful insights from data and improve patient outcomes.
- Modernization: Instead of attempting to offload legacy systems, healthcare organizations can link them together with DataOS by creating a modern operational layer. This layer enables organizations to reignite the value of legacy systems with minimal operational disruption.
DataOS is the key to unlocking real value from healthcare data. It enables non-IT users to integrate data seamlessly into decision-making, from patient diagnosis and treatment to organizational planning. It's the one missing element from today's healthcare technology stack; leveraging it will enable healthcare organizations everywhere to fully realize the power of their data.