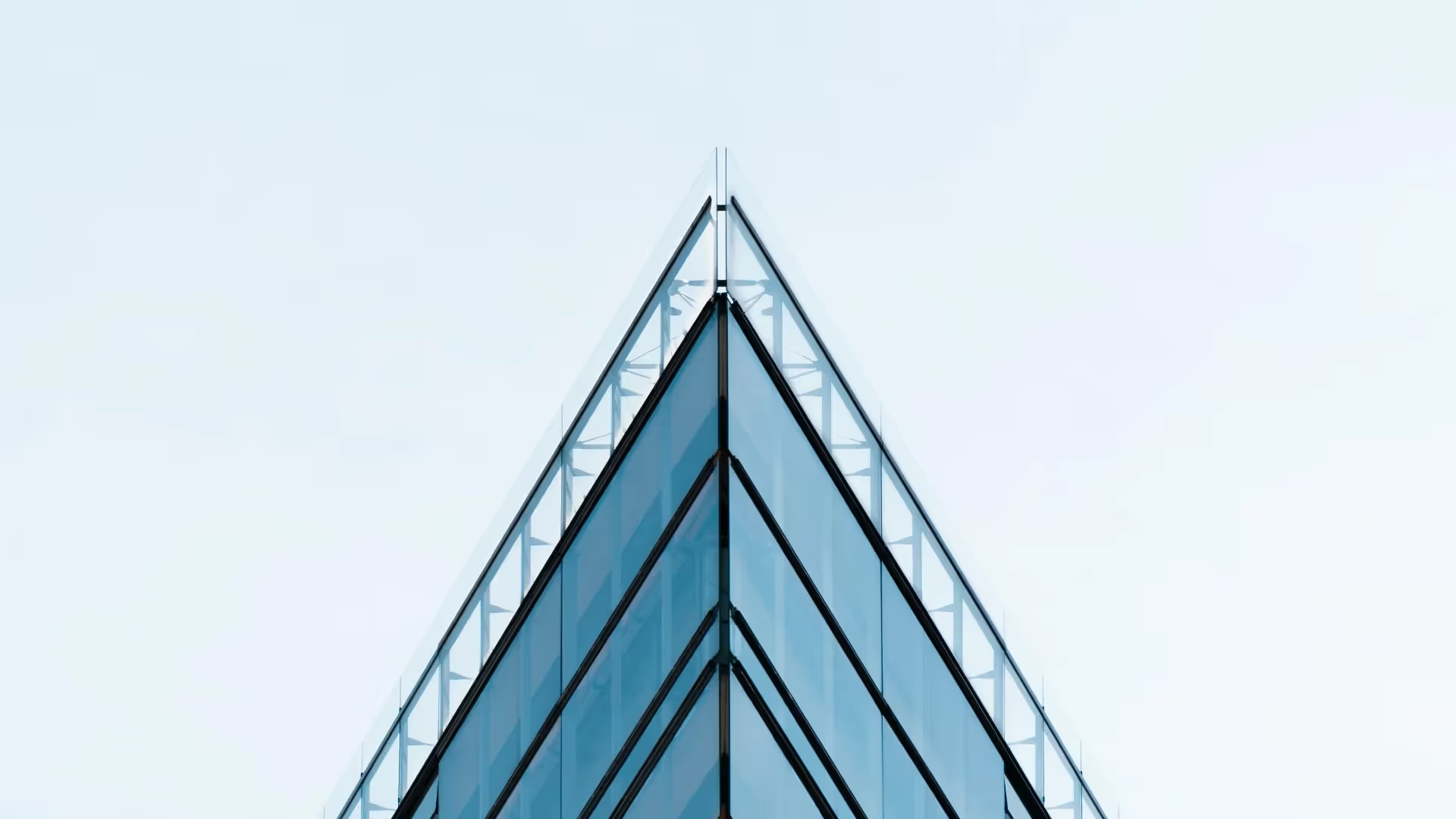
Data is healthcare’s number one asset and number one risk. With specialized compliance requirements and regulations for sensitive data, healthcare organizations are in the challenging position of having to both manage and make use of a wide array of powerful, yet sensitive, data.
In order to realize the potential of its data through analytics, the healthcare industry must fundamentally change the way it views data. The Modern Data Company Co-Founder, Srujan Akula, explained why in a recent interview that is summarized here.
The Evolution of Data Management: Data 1.0
In the early days of data management, there was heavy emphasis on ETL or “extract, transform, load” processes that took data from a source system and made it available for analysis within a different platform, usually a data warehouse. In this first approach — Data 1.0 — users transformed data before it ever entered a data warehouse. This process is labor-intensive because it requires pre-defining transformations to enable the data to be stored in an effective way in the receiving system. In the case of data warehouses, this was usually a variation on what is known as 3rd normal form.
The Evolution of Data Management: Data 2.0
ELT — or “extract, load, transform” — follows the same steps but in a different order. This second approach is Data 2.0. There’s no need for staging because data loads into a data warehouse or data lake in its raw form without any processing or transformation. ELT ingests raw data quickly. Then, thanks to expanding processing capability, the heavy processing required for the transformation stage occurs within the target platform itself. A key advantage to this approach is that it also allows organizations to handle unstructured data.
While the raw data lands quickly, most users and applications still must await the output of the transformation process. However, when fast access is required, the raw data can be accessed directly as well. The drawback to this model is that the raw data will not have the security and quality protocols applied and it will be incumbent upon the user or application accessing the raw data to apply any security and quality filters. This adds risk while enabling speed.
In the healthcare industry, the balance between speed, flexibility, and security is a difficult one. Relying on one-off transformations when accessing raw data is risky. At the same time, waiting for all of the transformations to be completed before accessing the data can take too much time. When data falls under strict regulations, such as HIPAA, and violations carry steep consequences, then the decision is usually made to prioritize security and accept the impacts to speed.
The Evolution of Data Management: Data 3.0
The healthcare landscape is in desperate need of digital transformation, but two significant obstacles remain in the way. Organizations cannot share data for training models if data security can’t be guaranteed, but the field cannot advance if it continues to take months to receive insights. How can healthcare move beyond a paradigm that relies on sacrificing either speed or accuracy?
The Modern Data Company has a solution: Data 3.0. This approach uses “model, map, load” to provide absolute security at a granular level while ensuring that data moves in real-time. It puts data into the hands of healthcare organizations’ team members, instead of relying so heavily on vendor solutions to do the work. The Data 3.0 approach can transform the healthcare industry’s relationship with its data.
Why Data 3.0?
The healthcare industry has a lot more regulation, compliance, and regulatory needs than most. These add a layer of security and governance complexity to an already complex data engineering challenge. The current typical approach, which uses the Data 2.0 paradigm, works to a certain extent. There are a lot of scale and security problems, however, and a lot of products today are not friendly to the requirements of high-frequency, high-scale data processing and operations.
Many data-focused products offered today are not focused enough on the operationalization of analytics. For analytics to be useful, it is necessary to build an operational layer attached to the analytical layer, to enable the analytics to scale and become useful. In addition, in the Data 2.0 approach, control lies too much with vendors versus the healthcare companies that want to operationalize their data.
Changing Healthcare Companies’ Relationship to Their Data
Data 3.0 is the modern way of thinking about data, where data becomes the connective tissue holding the organizations tools and requirements together. The concept of a data fabric has become popular. However, the way organizations like Gartner and Forrester describe data fabric makes it too oriented toward managing individual silos of data within a larger framework. The issues of integrating and driving value from the fabric are not as well addressed.
That is not how things need to be. It leads to too many point solutions and too much complexity. Whichever platform does the data management and data engineering for an organization also needs to have a layer that enables processes to be operationalized and made available to the business in the format the business needs and with the speed it requires. This approach, which takes the data fabric concept and adds a layer of operalization capability is the right way to approach data today.
To achieve Data 3.0, it is necessary to handle three major pillars:
1. Data Engineering
- Data engineering encompasses everything from data ingestion to building data pipelines. Today it’s usually ETL- or ELT-based and is still typically approached with a point solution focus.
- It is necessary to start moving towards more of the Data 3.0 approach of model, map, load (MML) in order to scale.
2. Data Governance
- If healthcare organizations want to truly use data in the modern Data 3.0 paradigm, it requires a governance engine that can support the necessary level of data operationalization and data scaling.
- Current governance products are too rigid and will not fully support a data environment in the healthcare industry where the data regulation and access has to be closely monitored and observed.
- Healthcare organizations need attribute-based access controls, not role-based access controls. They must apply data security and governance compliance at the most atomic level with flexibility in writing policies to use data.
3. Observability
- A lot of data products today are not transparent. Many are almost like a black box. You send data in, magic happens inside, and there is some output on the other side.
- That will not work from a risk and compliance perspective and it also will not scale. Being able to observe both the rules and their usage within the system is critical for success.
Healthcare Can Build a Modern Ecosystem with Data 3.0
The Data 3.0 approach discussed in this blog is what is needed to evolve to a modern data ecosystem because it shifts focus from data processes to data outcomes. Today, many healthcare companies are unable to focus on the outcomes of their data usage because they are spending too much time and effort focused on the processes. The three pillars discussed - data engineering, data governance, and observability - give healthcare companies the ability to extract more value from their data in a rapid and secure fashion.
The Data 3.0 vision has been productized as the DataOS offering from The Modern Data Company. To see how DataOS can transform your use of healthcare data to drive value, contact us to schedule a consultation.