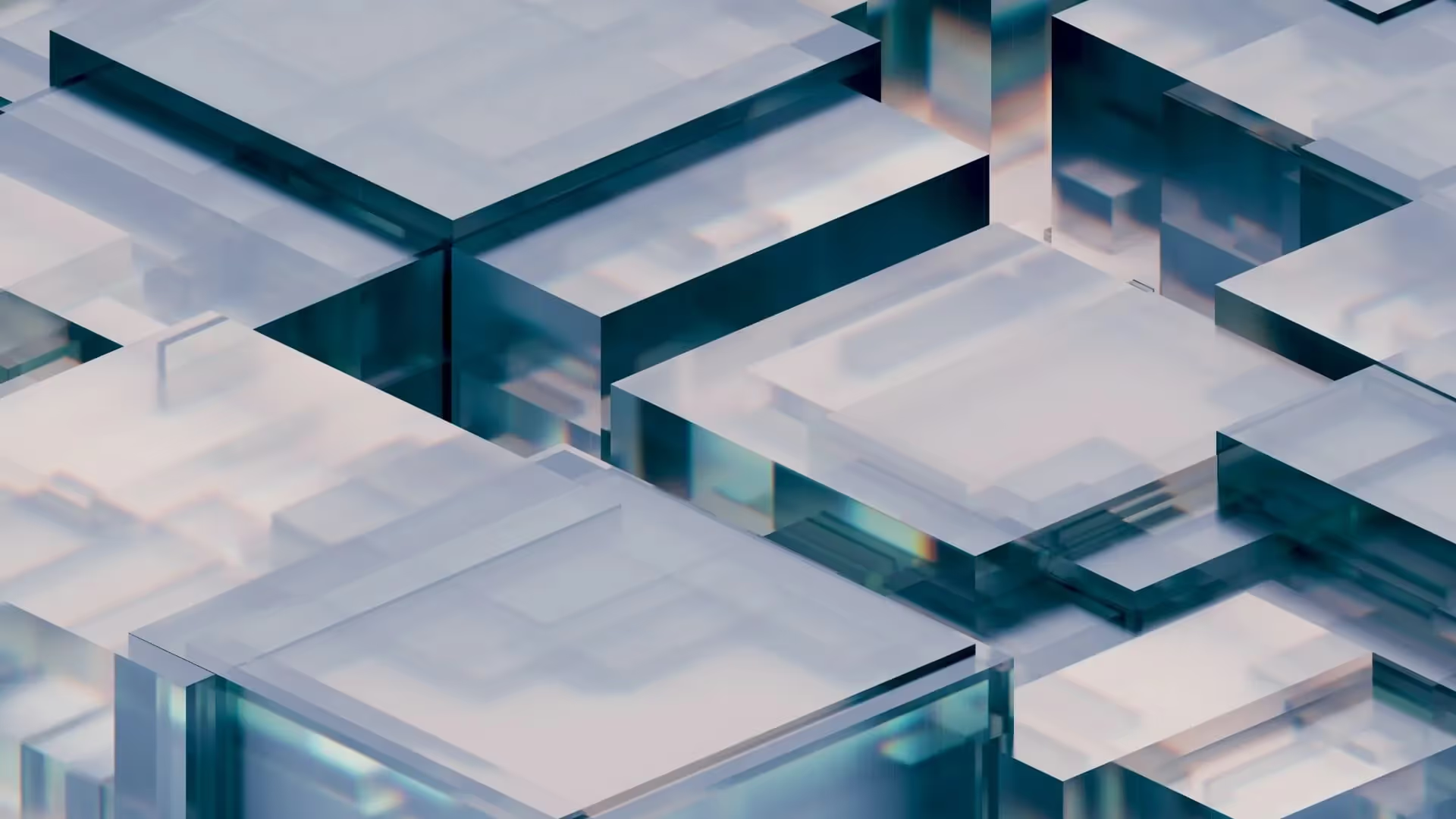
Data governance is on everyone's mind as we move from a static model of data to a dynamic, living, breathing data architecture. As many brilliant people have already said, data — and knowledge in general — is full of "ghosts," or implicit knowledge existing within any organization that never makes it to documentation. Ghosts affect companies in both data and people spheres, making it challenging to modernize legacy architectures or bring new talent on board. Even more, it makes using data a challenge.
Governance goes beyond security. It's a mission for data, a way of viewing and thinking about data. And shifting the governance paradigm will directly affect who makes it through the next hurdles of digital transformation. Let's explore what it's going to take.
The core elements of data governance
There's no need to discuss the benefits of data governance; there isn't anyone who would argue against it. However, data governance can look different depending on the organization.
Balancing security with flow
Data is only worth its insights, and users can't generate insights without data flowing into their analytic queries. The only problem is that the freer data is, the more likely loopholes are to occur. Companies must balance freeing up their data with ensuring it remains secure and compliant with any privacy regulations.
Companies that can create rules based on user attributes are able to offer data to all stakeholders but in a compliant format. For example, someone in legal may be able to see all rows and columns for the latest reports on those in the mortgage process, but marketing will receive data tables with sensitive information like names scrubbed out.
Addressing the ghosts
Another component of data governance is transparency. Companies must determine data lineage and data quality through consistent and scalable methods. This requirement means deactivating silos so that administration has a clear view of what data is being used, where it came from, and its context. Knowledge around topics like lineage and context are simply too important to exist only as implicit ghost knowledge. The information must be explicitly documented and available to all.
From there, data quality is the next step. Data quality is the enterprise's plan for what to do with data before making it available — for example, removing duplicates, ensuring accuracy, and working toward completeness. Then, nontechnical users will be able to query data and receive quality results.
One specific way to address these ghosts is to remove complexity, i.e., the inherent structure behind the data. Instead, a system that requires as little of this implicit knowledge as possible helps ensure that conclusions reached by nontechnical users are sound. The system would speak the user's language rather than forcing the user to speak the language of machines.
Addressing stewardship and business value
There's no globally applicable standard process to clean data and no standard checklist for ensuring data is ready for use. There are best practices, of course, but ultimately, each enterprise has to put its own policies in place. Data stewardship should be part of a well-rounded governance plan to ensure consistency with these internal policies, and data stewards appointed to serve as the expert responsible for applying and monitoring those policies.
Data stewardship is about enabling businesses to define and implement policies ensuring that data is usable, accessible, safe, and trusted so that team members can rely on insights to make critical departmental decisions. In addition, stewardship should align all data initiatives with clear business value to bridge the gap between the data side and people.
As the key overseers of that stewardship, data stewards fill a vital role in a governance structure by acting as subject matter experts for the data domain. They ensure data usage is applied consistently and accurately across any reports or systems. They also work with departmental experts to standardize processes and governance procedures across the enterprise.
A Data Operating System enables granular governance controls
The sheer scope of data volume makes governance tricky to apply consistently across the entire enterprise. Add in legacy systems or tools and data acquired through mergers or acquisitions, and governance can be a monster to implement.
A data operating system provides a single connective tissue for all data, tools, and systems present in the entire architecture. Administration and decision-makers can view the ecosystem as a whole to determine critical governance questions such as:
- Who is using data and why?
- Where is data located and stored?
- Which business and risk processes does data impact?
- What dependencies exist downstream?
The operating system also provides granular governance controls so that administration can open data to flow to stakeholders without risking privacy or security loopholes. Users can search for and see what data is available for use, while stewards can see who is using data, when, and why.
An operating system is the key component of a modern governance strategy. It enables consistency in governance implementation—something nearly impossible with piecemeal, siloed tools and solutions.
DataOS will shift the governance paradigm
The Modern Data Company has built a data operating system, DataOS, to unite technical and nontechnical users across the enterprise. With right-to-left engineering and simple search functions, nontechnical users can find the data they need and build their query or report without needing to depend on IT. In return, IT can use a standard command-line interface to build higher-order, repeatable pipelines, and processes that move the needle for business value.
To discover more about how DataOS can transform the way companies implement governance and data access, download our white paper: “Data Security and Governance in DataOS”.