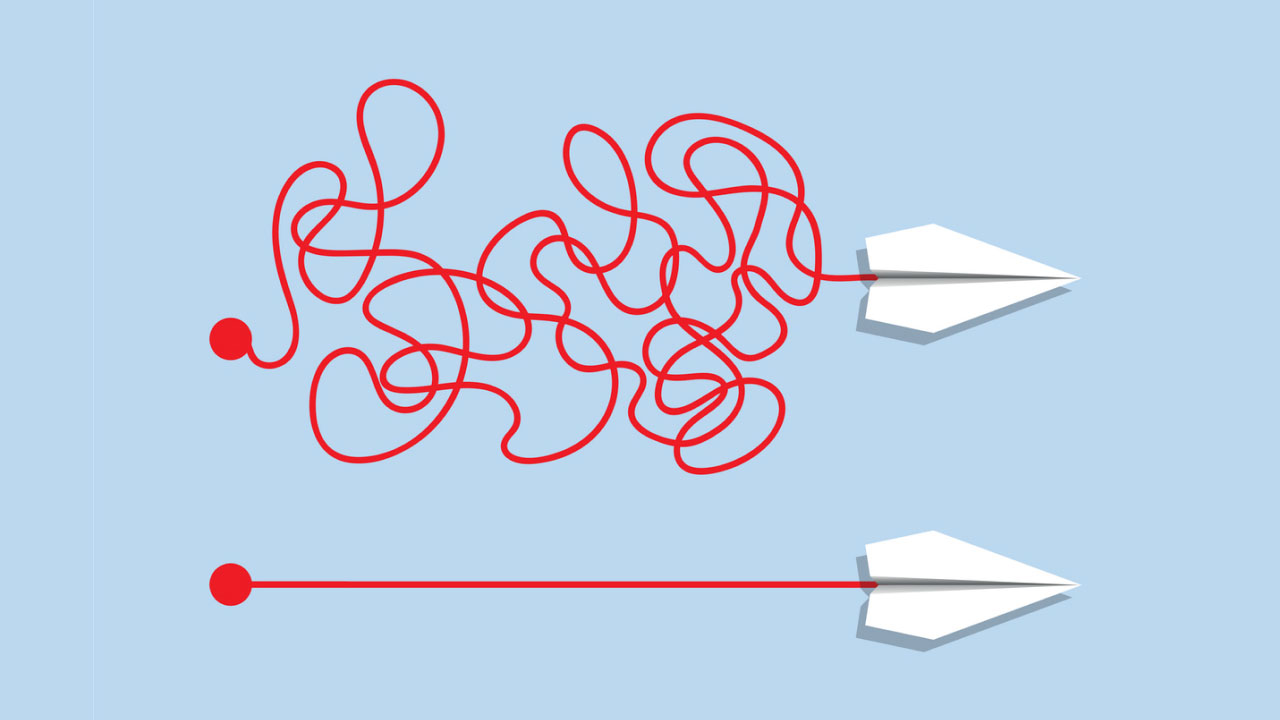
TLDR: Modern Data's approach to data products demonstrates that purpose-built, lean solutions for data beat massive, complex ones. Regardless of your views on DeepSeek's AI models it rejects the "process everything" mindset by targeting specific outcomes and focusing resources-parallel to the data product philosophy.
The Traditional Approach: More is More
For years, the prevailing wisdom in both data management and AI has been to process everything, store everything, and use massive resources to handle it all. Traditional enterprises build sprawling data stacks with ETL pipelines, data lakes, and numerous specialized tools. Similarly, leading AI companies like OpenAI have achieved their results by deploying more GPUs to the problem–relying on enormous datasets, ever-larger models, and expanding computational resources.
This "boil the ocean" approach has led to:
- Massive infrastructure costs
- Complex tool sprawl
- Long delays in delivering business value
- Heavy reliance on cloud resources
- Significant technical debt

A New Paradigm: Efficient, Purpose-Driven Solutions
Rethinking a domain from first principles, whether it’s AI or the data that feeds it, shows there's another way. Modern's approach to data products and what we know about DeepSeek's AI model share some similarities in their fundamental approach:
Focus on Outcomes, Not Outputs
An implementation of Modern’s DataOS platform starts with specific business outcomes and works backward, typically needing only 15-20% of total enterprise data. Similarly, on some level, DeepSeek shows us that AI models don't need massive compute resources to achieve impressive results - matching GPT-4 level performance at just 5% of the computational cost. Their approach uses techniques like test-time scaling to achieve better outcomes with fewer resources.
Innovation-Driven Efficiency
For large, global enterprises, Modern's data product framework has achieved:
- 70% reduction in data processing volume
- 60% fewer tools required
- Weeks to value instead of months
- Up to 50% reduction in Total Cost of Ownership
Meanwhile, DeepSeek demonstrates that smaller, distilled models can often outperform larger alternatives, setting new standards for compact AI performance. This efficiency isn't achieved through brute force, but through innovative approaches like reinforcement learning and strategic model distillation.
Democratization of Capabilities
Just as DataOS is making enterprise data management more accessible by reducing complexity and cost, DeepSeek's breakthrough could further democratize AI development. As noted by AI researcher Ritwik Gupta, "If the goal is to get everyone to have their own 'personal AI', then it will be necessary for small models to run on people's personal devices."
Implications for the Future
This parallel evolution in data and AI points to a broader trend in enterprise technology: the move away from bloated, resource-hungry solutions toward more efficient, purpose-built alternatives. The implications are significant:
For Enterprises
- Reduced dependency on massive cloud infrastructure
- Predictable operational costs
- Faster Experimentation
- Greater flexibility and control
For Innovation
As chief architect at Modern Data, Travis Thompson notes, "DeepSeek's milestone shows us that innovation drives efficiency–doing more with less. This principle will reshape the world of data, where working within constraints consistently sparks breakthroughs. Expect to see the efficiency revolution accelerate further over the next 18-24 months."
The Road Ahead
We're at the beginning of a fundamental shift in how we think about enterprise data. The future belongs not to those who can process the most data or deploy the largest models, but to those who can achieve the best outcomes efficiently.
This shift promises to make both data management and AI more accessible, and aligned with business needs. As these technologies continue to converge, organizations that embrace this new paradigm of purpose-driven solutions will be best positioned to succeed in the AI-driven future.
What can help organizations move through their data and AI journey faster is a data product approach. To learn more about how DataOS can accelerate your data transformation, schedule a demo of DataOS today.