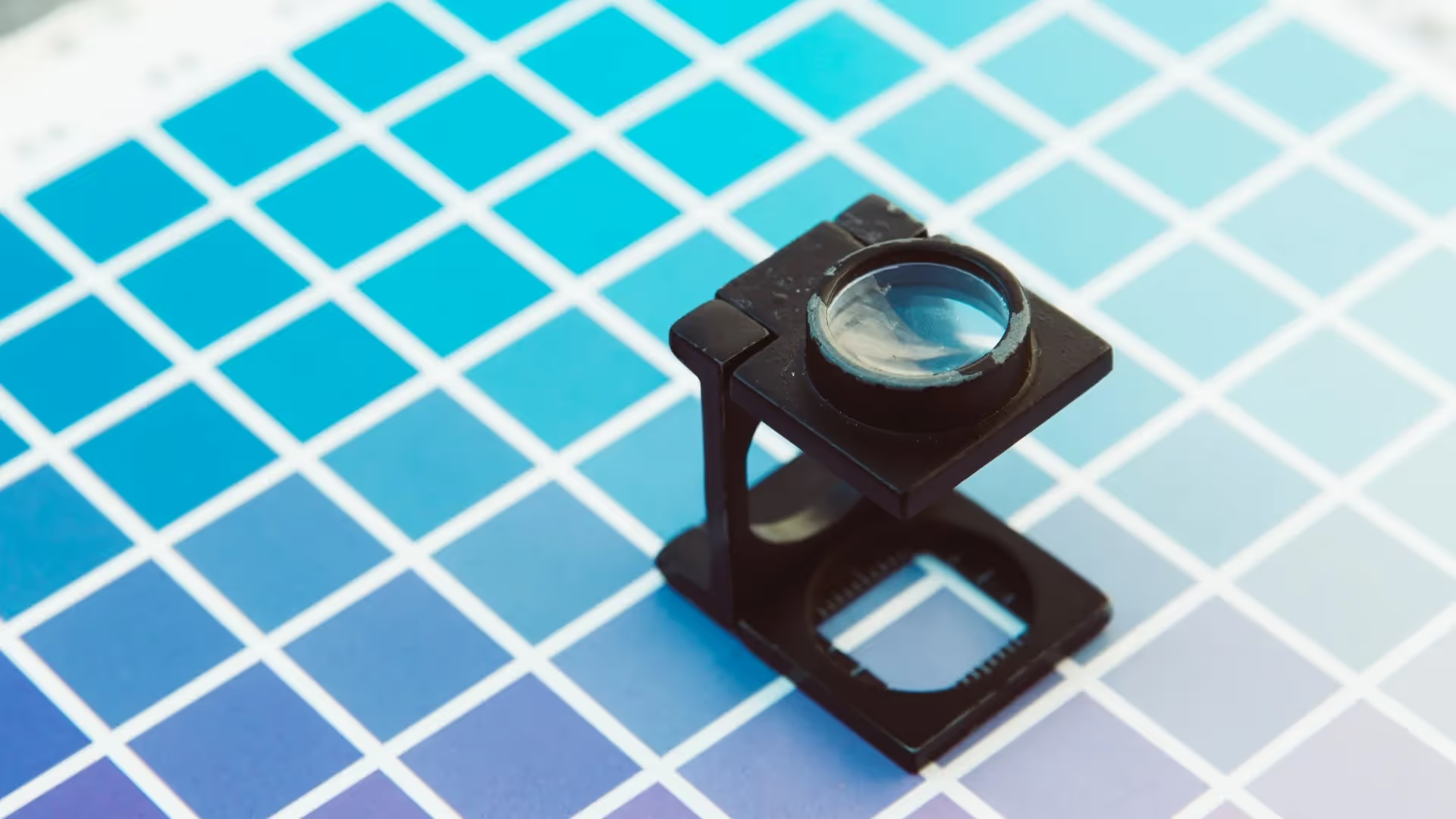
Companies capture data like champions, but there’s always a sneaking suspicion that they aren’t using the data to its fullest potential. Unfortunately, this isn’t just the companies’ imagination (or paranoia). A startling amount of data goes totally unused, not simply used in a less than optimal fashion.
So what’s happening? Inconsistent formats, out-of-date datasets, and silos all over the place are preventing companies from becoming data-driven. A lot of unused corporate data does have value, but it isn’t easily accessible for business users and so they don’t make use of it. Of course, plenty of the data that is actually used is not used to its full potential, either. All of this puts in place barriers to the adoption of a data-driven culture. One way to make significant headway is to engage in regular data health checks.
The first place to start with data health is the quality of data. In this blog, we’ll focus on five specific quality checks that can be implemented in order to drive adoption and usage of data higher while enabling more value to be generated from the data.
Health Check #1: Is The Current Data Complete?
The first and simplest check is to validate that every data source has the full amount of data it is expected to contain. For example, if a transactional data source is expected to have all transactions from 1/1/2015 through today, is the data all actually present? It isn’t at all uncommon for certain periods of data to be missing, whether because someone forgot to update it or because an update process failed. Nothing frustrates users more than data they expect to see not being available. Missing data not only corrupts results, but it lowers user trust.
Health Check #2: Is There New Data To Add?
New data sources become available all the time. Just because a platform contained all relevant data a few months ago doesn’t mean that it still does today. There might be new customer survey data, or new sensor data, or data from a new mobile app. Whatever the case, it is important to identify new sources and if they are valuable enough to make available to users for analysis purposes. If so, then the data should be loaded and an process put in place to regularly update it moving forward.
Healthcheck #3: Is The Data Timely?
Some data is generated and used infrequently, while some data is generated and used in near real-time. Any data source must be updated at a pace that matches the business requirements. Some data, like demographic data, changes rarely and requires only infrequent updates. Other data needs to be updated much faster. For example, perhaps a transaction file available for analysis is found to be current as of the previous day. That sounds great unless the file is supposed to be updated every 5 seconds in order to facilitate website customization. There is no absolute definition of timeliness as it relates to data. Rather, every data source must be “timely enough” for its intended uses and purposes.
Healthcheck #4: Is The Data Accurate?
Data quality is one of the biggest issues corporations struggle with and it is one problem that is never fully solved. Even if all historical data is certified today as being 100% clean and accurate, that can change rapidly as users or applications update pre-existing data or add in new data that contains errors. Accuracy starts with data ingestion procedures being constantly updated to fix problems that have been identified. It is also a best practice to continuously monitor the distribution of any data element to identify when something suddenly looks different. All the right values of transaction type might be present, for example, but in all the wrong proportions. Automating basic data accuracy checks is necessary in a data-driven environment and it is becoming a common approach that is being implemented broadly.
Health Check #5: Is The Data Enabled For Performance?
Data not only needs to be available, but it needs to be available in such a way that users and applications can query and analyze it fast enough for their needs. Simply dropping raw data into a platform and turning people loose on it is not a winning formula. Even when a data platform has been tuned and optimized, settings will need to be updated. Usage patterns of the data within the platform can change. New data sources can become very popular. A large number of new users or processes might come online. Any of those can make what was a high-performing platform start to struggle. It is necessary to constantly monitor and track performance and be ready to make adjustments over time as change occurs in how the data is accessed and used.
Summary
Of Course, data quality isn’t the whole story. Companies must also consider other areas such security and governance for a complete picture of data health. Those may be addressed in a future blog. Making the health checks discussed here a regular practice provides a solid foundation for finally using all — yes, all — available data effectively and efficiently within a data-driven environment.
One approach to a data fabric that can enable everything discussed here has been productized as the DataOS offering from The Modern Data Company. To see how DataOS can transform your use of data to drive value, contact us to schedule a consultation.