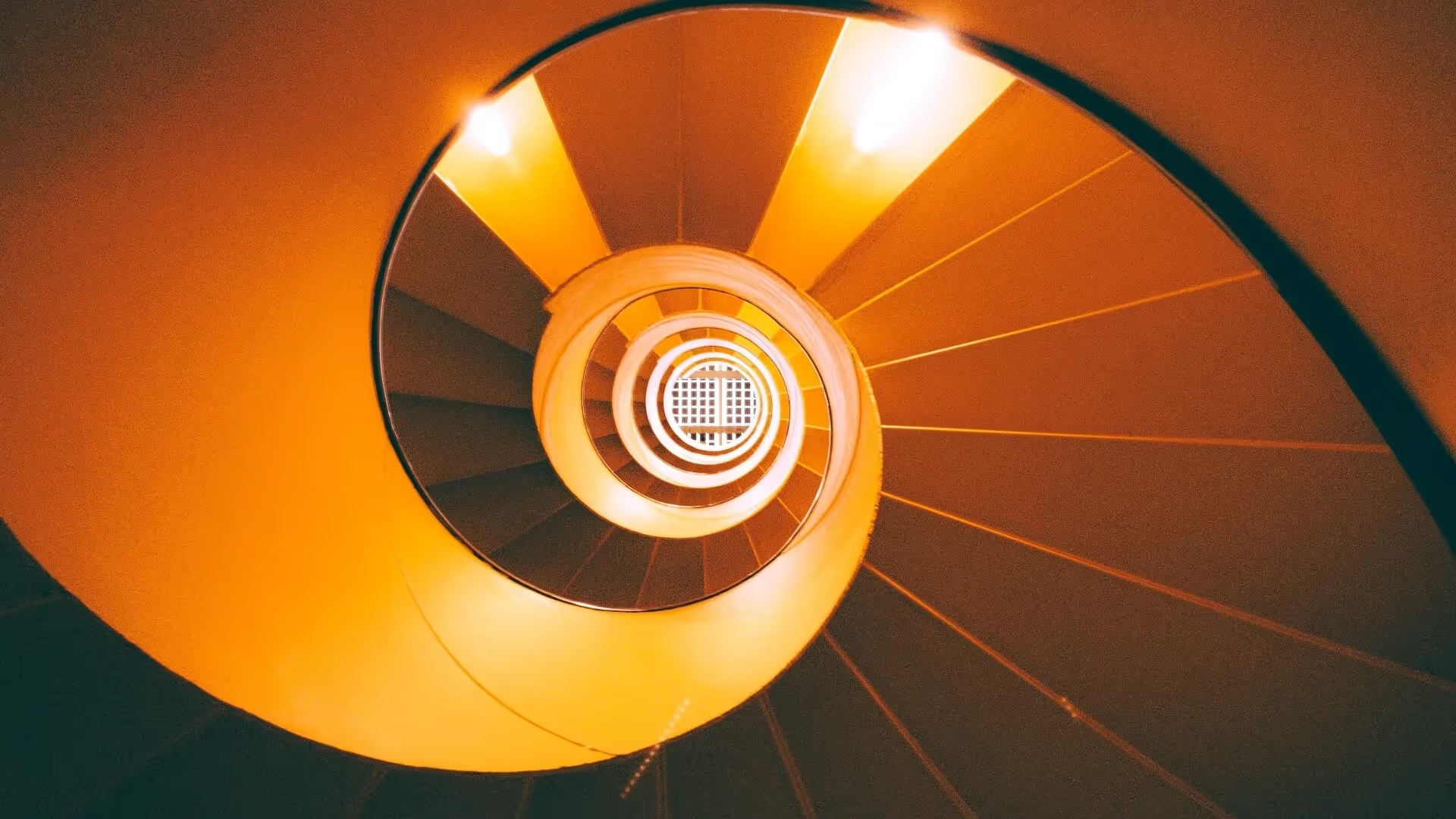
Inaccurate data affects the bottom line of 88% of organizations, leading to an average loss of 12% of revenue. This loss of revenue comes from insufficient data to inform and drive business goals. Data quality starts at the source — which is why it’s so important how data is selected, sourced, and moved.
Data sourcing is the process of extracting and consolidating data. This data can come from both external and internal sources. Collectively, these data sources make up an organization’s data infrastructure. This is a prerequisite for data to be properly activated so that it can be used in various workflows and help to achieve business outcomes.
There is not just one type of data source; it can be a database, local file, matrix stored on the cloud, an app SDK, web application, or any of the multitudinous digital data services. It’s also important to keep in mind how integrations relate to one another and the needs of various data consumers for a given source to avoid a frenzy of redundant data pipelines.
How can you ensure that your data is valuable, trustworthy, and properly protected? It all starts with data sourcing. It comes down to what data you choose to source, how and where the data is moved, the rigor put into quality efforts, and schemes in place for ongoing management and maintenance of the data supply. Ultimately, since the source is the foundation of data quality, it is therefore necessary for all other data-driven initiatives.
Steps for sourcing quality data
Clarify the specific goal
Data is only good or bad insofar as it helps achieve business outcomes. That’s why it’s essential to start with the end target or specific goal that sourcing this data will help you achieve. Sourcing should actually start with speaking to the data consumer to understand their needs and priorities. The business outcome will help to determine all of the following steps of data sourcing.
Review the current infrastructure
Getting to know the data that is currently being sourced for a team and the pipelines that are being used are the next steps in the process of data sourcing. This is critical to avoid duplication and creating additional integrations that can lead to an increase of failure points and potential vulnerabilities. It can also help illuminate what is or isn’t working for the data consumers and get information about the data they need.
Meet the data owner
It isn’t always easy, but meeting the data owner is an essential step of the data-sourcing process. Those who own the data are not necessarily interested in allowing it to be sourced for other departments and objectives. That is because it can create more vulnerability and risk. By combining metrics about the predicted business outcome metric and an understanding of the current infrastructure and projected usage information, you can make a compelling argument for accessing the data.
Once they have agreed to share the data with you, there are still a number of additional clarifications needed. It’s key to ask the data owner about the quality of the data, the refresh frequency, expected format of the data, and any additional fun facts about the data — like a unique hash of the customer ID.
Select a lean data set
Most data consumers, especially at organizations where data democratization hasn’t been achieved, will take all the data you can source. However, this is not the best approach. A lean set of data should be selected to ensure quality checks are properly put into place and that the data “pays its way” by helping achieve a business goal. Priorities should be put on data based on a given data element’s estimated impact or necessity to the goal.
Identify quality issues
Since data is often manipulated in various ways as it travels throughout the data infrastructure, it's best to catch data quality issues early. According to the 1-10-100 rule, data becomes exponentially more expensive to fix depending on its stage. This makes data sourcing the ideal place to catch any data quality concerns. The data consumer should be brought back in at this stage to help evaluate the quality of the data. Depending on the department, they will likely have an idea of expected ranges and be able to support with quality assurance.
Plan for change
The only constant is change: data needs change, business priorities change, products change, and customers change. That’s why it’s imperative to have a change management strategy in place from the beginning. You should set expectations for maintaining the current data sources, dealing with any issues that arise, and managing future requests as needs and business goals evolve. Setting these expectations before building the extraction and integration of data will streamline future work.
The future of data sourcing
Modern data tools like Modern’s DataOS support the data sourcing process from end-to-end. DataOS was developed to support left-to-right thinking, powering a business-driven data infrastructure. With this tool, you’ll have full visibility of the available data, helping you to locate the correct data for your need. Wherever it lives, you’ll be able to see the data owner, the quality rating of the data, and where it is located — exponentially speeding up these essential first steps.
Whatever the source, it’s essential that your teams have the right tools to ingest and activate the data. DataOS offers an out-of-the-box data ecosystem that includes native compliance and governance. Data is freer when it’s more secure, allowing you to source the data you need without exposing it to additional risk.
Want higher quality data that drives business outcomes? With DataOS, you can easily locate, extract, and operationalize data throughout your entire organization — starting at the source.