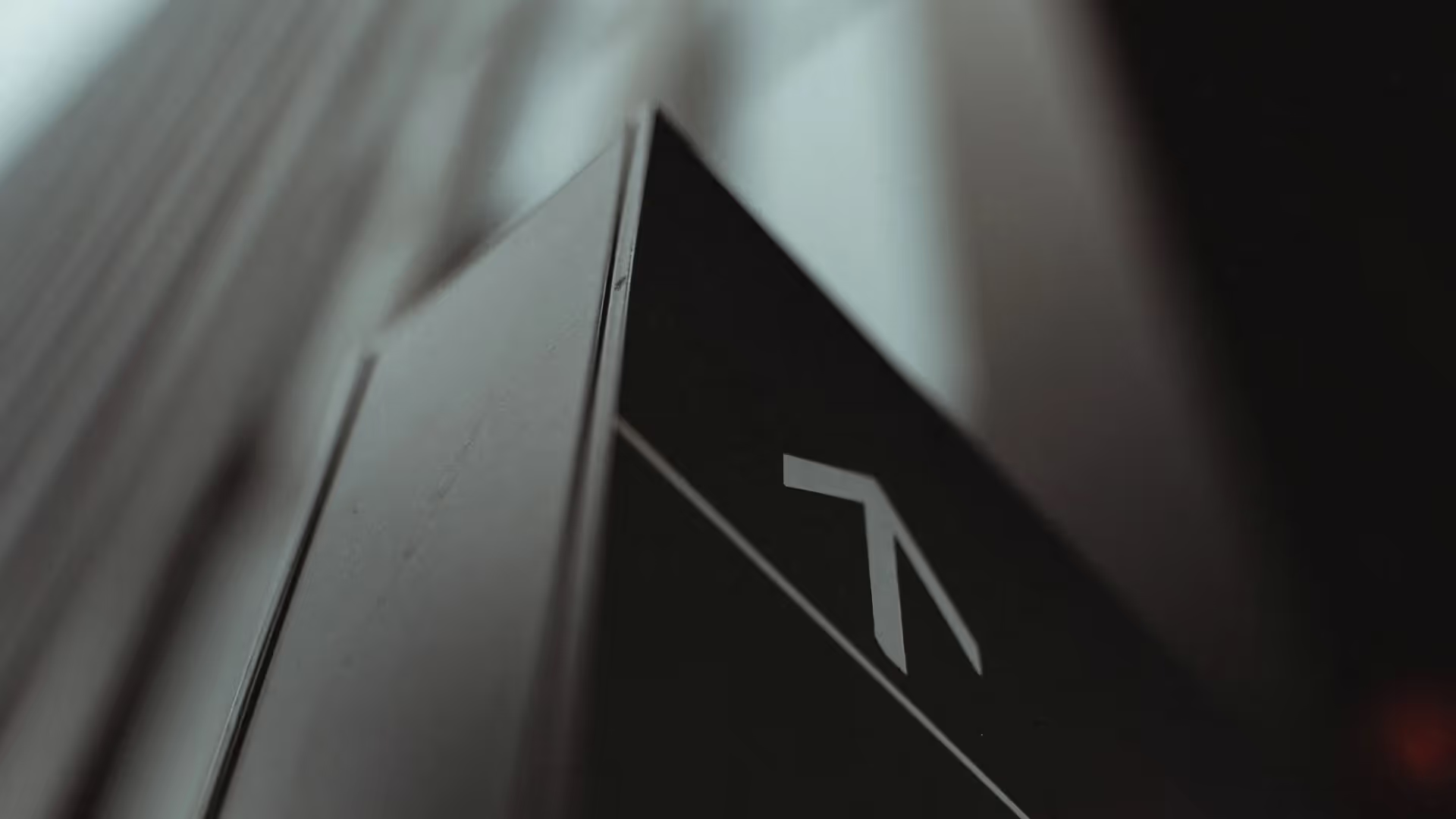
When discussing self-service tools for data and analytics, people usually place their focus on the goal of enabling non-experts to do more things for themselves. While enabling citizen data scientists, citizen data engineers, and others to do more with data and analytics is a noble goal, there is another perspective to consider. The same tools and functionality that experts implement to democratize data and enable non-experts to do more can simultaneously be used by experts to increase their own productivity. This increase in expert productivity has the potential to drive a massive return that is often not recognized or pursued.
The Traditional View of Self-Service
The traditional view of self-service is that automated modeling tools or streamlined data mapping tools are best targeted to non-experts. The tools are used as a way to enable non-experts to do more for themselves and to free up experts to focus on other things than supporting users’ basic needs. The concepts of citizen data scientists and citizen data engineers arose from this approach. It isn’t an approach without merit, but it also has risks.
Unless the tools are tightly managed and usage is tightly governed, there is a risk that these citizen scientists will use the tools to do things that are incorrect. Worse, the non-expert won’t be able to identify that there is an issue due to their limited depth of technical knowledge. For example, it is common for a non-expert to build a predictive model using a point-and-click tool without realizing that their problem is ill-formed and that the data they are feeding the model is not appropriate for their needs.
Managed well, self-service tools can largely minimize or avoid the risks mentioned while enabling more people to perform data and analytics tasks. The traditional view of enabling citizen data scientists and engineers is valid and achievable, but it isn’t the only path to value.
An Additional View of Self-Service
The same tools that are implemented to enable non-experts can also help reduce the workload and increase the efficiency of experts. This is because an expert can use the same point-and-click environment to get work done faster. For example, a data science expert knows how to properly define a model and how to feed the correct data into that model. Instead of coding a process manually, a self-service tool can be used to expedite the process. Similarly, a self-service data pipeline tool can be used by a data engineer to create a new pipeline more quickly.
The important point to recognize here is that experts want to streamline their efforts and be more productive just as much as non-experts do. If self-service tools that are often aimed at non-experts can also be used by the experts themselves, then that’s a huge bonus! The reality is that experts will be able to fully understand the pluses and minuses of a self-service tool and then begin applying the tool very quickly. The experts will also be able to push a self-service tool to its limits and learn what needs it best matches. Having the experts test drive a new self-service tool before releasing to a wider audience is a great way to not only drive extra value from the experts but also to develop a plan for effectively rolling it out to the non-expert users.
Maximizing the Value of Self Service Tools…For All Users
To get the most from self-service tools, it is necessary to have access to a broad range of data and to have the tools plug into existing governance mechanisms. Is there a way to make this easier? Yes! A modern data operating system, like DataOS from The Modern Data Company, provides the underlying platform that can enable successful rollouts of self-service tools to experts and non-experts alike.
A data operating system connects to all source systems, whether new or legacy, and makes a single view of all corporate data available. Self-service tools can point to this layer to provide a user with visibility into all the data the user has permission to use. Of course, security is important, and a data operating system can apply finely-detailed permissions so that any given user can only see and access the data they are allowed to see. By defining permissions once in the data operating system layer, the security settings will cover all downstream applications seamlessly.
When users request an analysis, the data operating system then coordinates the data requests to the various underlying systems where the data resides. It will compile the results and feed them back to the application that requested it. Just as a self-service tool allows experts and non-experts alike to get more done with less code, a data operating system makes it much easier to access and govern a wide range of data sources. When a data operating system is combined with a self-service tool, there is speed and efficiency gained across all aspects of an analysis. That’s a strong value proposition worth understanding and exploring.
To learn more about how a data operating system like DataOS can help your organization democratize data and enable more self-service, download our white paper, A Paradigm Shift in Data Management.