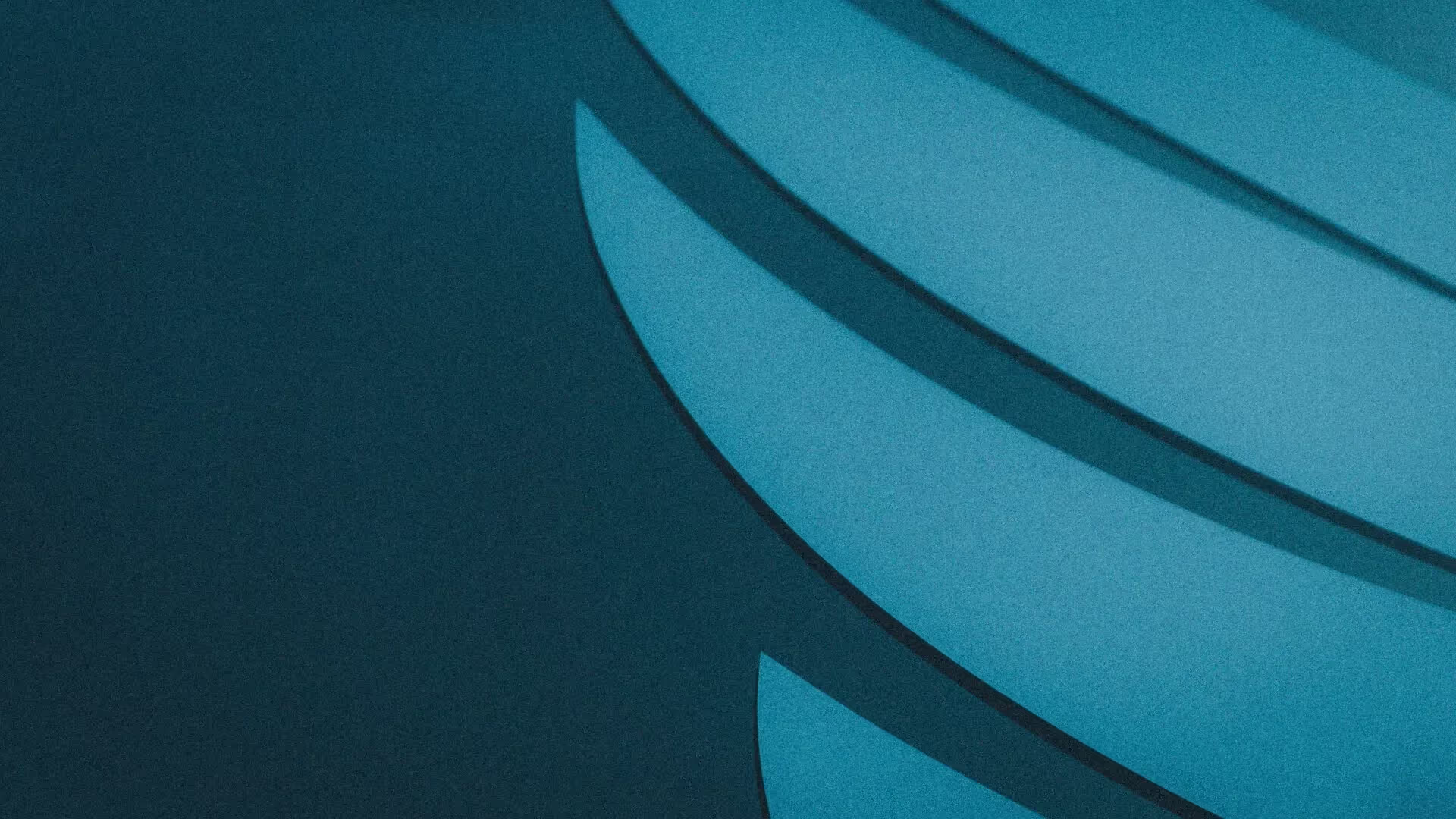
The Case of Twitter
Twitter handles around 500-700 million tweets per day, equating to roughly 12 terabytes of data every 24 hours. Another way to understand the scale is to consider that this amounts to over 4K terabytes of data each year. Even with an army of the savviest data scientists and engineers, handling this much data is a challenge. And adding to this challenge is the fact that much of the data is freeform text, albeit short text, which is stored as unwieldy unstructured data.
And then there are bots. This means that engineers are essentially tasked with creating machines that can pass the Turing test — and coming up with supplemental approaches. As Parag Agrawal, the CEO of Twitter wrote, "Spam isn’t just ‘binary’ (human/not human). The most advanced spam campaigns use combinations of coordinated humans + automation.” Agrawal added, “fighting spam is incredibly *dynamic*…You can’t build a set of rules to detect spam today and hope they will still work tomorrow. They will not.” Every day, Twitter suspends over half a million accounts and locks millions of accounts each week that are suspected of being spam. Meanwhile, this has to be balanced with avoiding suspending or adding excessive friction for real people — which can be troublesome given that many real accounts may superficially look fake.
Now add to this the complexity of creating reporting on spam accounts that is both up-to-date and accurate. Reporting like $44 billion depends on it — because it just might. Earlier this year, Elon Musk offered to buy Twitter for $44 billion. Afterwards, he tried to back out of the deal, claiming that Twitter was infested with a larger number of “spam bots” and fake accounts than they had disclosed. Twitter retorted that they use a “generic web tool” that classified even Musk’s Twitter account as a possible bot.
Twitter estimates <5% of reported monetizable daily active users (mDAU) per quarter are spam accounts. They come to this estimate using multiple reviews from humans replicated over thousands of randomly sampled accounts continually over time from accounts that are considered mDAU. Agrawal emphasized that combining both private data and public data is essential to accurately determine whether an account is spam or real. It goes beyond a Twitter handle that is NameAndABunchofNumber with no profile picture and unusual tweets. Important data points include IP address, phone number, geolocation, client/browser signatures, and what the account does when it’s active. Even these are part of a higher-level description. These estimates are done each quarter, and according to Agrawal, they have small error margins.
What Can We Learn?
If you deal with data in your day-to-day, then you likely understand the scale of what was just described. There are many key topics embedded in this case:
- Disparate data types that need to be visible, understood, and centralized
- High-skilled time spent on creating and executing processes for accurate reporting of business problems
- Handling massive amounts of structured and unstructured data that necessitate trustworthiness for business, technical, and machine users
- A business need that relies wholly on data availability and accuracy
- The demand to use and build accurate AI/ML models on an expanse of complex data
To produce an accurate rebuttal of Musk’s accusations, Twitter needed to present accurate processes for dealing with the identification of spam bots. This involves acquiring, managing, and governing data that is both structured and unstructured and public and highly sensitive. This is not an easy task.
When it comes to solving these challenges, many organizations get stuck in data swamps. Often, this means that 90% of time, energy, and cost is spent on moving, selecting, sourcing, synthesizing, exploring, cleaning, normalizing, and tuning data. This leaves only 10% of time to extract and generate value from this data. Armies of data engineers juggle a spaghetti of ETL’s using Airflow, Python scripts, and Talend, which require constant monitoring and maintenance.
This 90/10 split of time, energy, and cost often results from systemic non-unified data infrastructures. Organizations’ data landscapes have become increasingly fragmented, and as a result, overly rigid. The rise of point solutions adds to this complexity and makes centralized and native governance, as well as observability, nearly impossible. Engineers, who would otherwise be building the intelligent systems like Twitter’s spam identification, must spend most of their time performing the duties of system integrators. This is because the traditional approach many organizations take focus on data processing rather than activation.
The case of Twitter demonstrates the powerful combination of business-driven data initiatives executed using an infrastructure that can fluidly combine necessary, trustworthy data points. Organizations like this that are truly data-driven have insight into available data, the ability to compose data, and the flexibility to work right-to-left. This allows them to meet complex business problems like spam bots with reliable data solutions.
What If You’re Not Twitter?
Not every organization is Twitter: we don’t all have an army of data engineers and scientists who can seemingly magic data into dreamy, exquisite AI/ML models and high-confidence quarterly reporting. That’s why our team at Modern built DataOS.
DataOS is a modern layer over your legacy or modern data infrastructure. This allows you to instantly use your data in modern ways without needing to disrupt your business. As soon as DataOS is implemented, you’ll get deep insights into all of your available data—no matter how dark or siloed. From there, most data analysis can be done while the data stays in place. That means you can move only the data that needs to be operationalized. Since DataOS was built with principles of outcome-based engineering, it can automatically retrieve data based on the need defined by business users, pipeline-free. The fully composable architecture of DataOS enables your organization to realize data fabric, lakehouse, CDP in weeks instead of years.
DataOS can superpower data initiatives across your organization, to help you realize a data-driven future and solve essential business problems—simply. The case of Twitter demonstrates the importance for your organization to operationalize disparate, siloed data sources to create accurate business metrics. This can be worth more than $44 billion: it means staying competitive in an ever-changing landscape.
Want to learn more about DataOS, and how it can help Twitter-ify your data? Download our white paper, DataOS: A Paradigm Shift in Data Management.